Frequently Asked Questions
Explore the Most Asked Questions
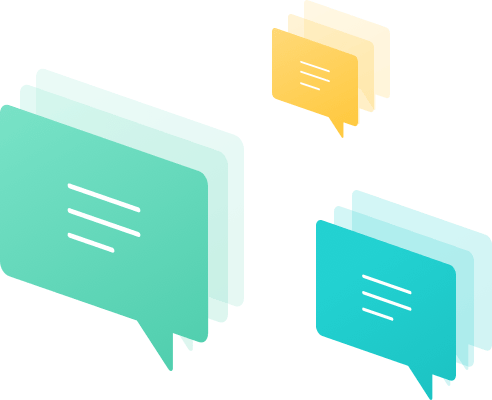
Clear
All
Product
Pricing
Integration
What is a recommender system?
A recommender system is a technology that is deployed in the environment where items (products, movies, events, articles) are to be recommended to users (customers, visitors, app users, readers) or the opposite. Typically, there are many items and many users present in the environment making the problem hard and expensive to solve.
Check our blog post explaining the topic in further detail here.
Check our blog post explaining the topic in further detail here.
How does Recombee tackle the “cold-start” problem?
By default, content-based models (text processing, image processing, and other types of meta-data) are turned for the cold start user or item with no prior interactions. As soon there is at least one interaction from the user or with the item, personalized models start to being used. This way our solution seamlessly tackles the cold-start problem.
Do you improve your product continuously?
Yes. We maintain a priority list of new features based on customer suggestions. We are quite strong in research, innovations, and inventing new functionality is our passion. Check out our articles on the Recombee blog to find out more about recently launched inverse recommendations and other features.
Is Recombee solution able to create personalized recommendations for new or anonymous visitors?
Yes. Definitely.
Anonymous users are very important and Recombee is built to learn from them and adapt in real-time as they make interactions. You can use whatever unique identifier for userID, such as the session ID, or GA clientID. You can learn more here.
Anonymous users are very important and Recombee is built to learn from them and adapt in real-time as they make interactions. You can use whatever unique identifier for userID, such as the session ID, or GA clientID. You can learn more here.
Can I assume that the data uploaded is not to be shared with third-party entities?
We never share data with third parties, neither we share data between individual clients (there is no cross-database data enrichment).
Does missing data in an item affect the recommendations?
It’s fine to have missing fields if the items do not have such properties by their nature. These items should be still recommendable.
Do item properties play a role in what recommendations are returned?
Yes, they do. Recombee solution is composed of a mixture of models, including collaborative filtering and content-based models. While collaborative filtering has a higher weight/priority, some of the recommendations are generated with the help of the content-based models (based on the item and user properties).
When are the collaborative filtering algorithms used?
Collaborative filtering algorithms are used when a large portion of the items is covered with interactions (having at least tens of interactions).
Do you track our customers/users, or do we have to do this by ourselves?
Tracking users is up to you. We only require a unique string ID for each of the users. Please note, Recombee is fully GDPR compliant.
Does Recombee have the ability to produce recommendations based on both the current user’s historical data (e.g. purchases, interests) and the demographic data applied as properties to the user (e.g. age, gender)?
Besides item properties and user interactions, user properties can be also used for producing recommendations. Sending user properties is optional. User properties are used mainly for new (cold-start) users without any previous interactions. When the user has interactions, the results produced by more sophisticated collaborative filtering models are preferred as they usually have better performance.
In what way does Recombee take into consideration of a user's past behavior. As in, items that are recommended but not clicked, or are clicked but not purchased. Are they taken out/reduced in future recommendations for that user?
They are taken into consideration according to the rotationRate and rotationTime parameters of the recommendation requests.
Does Recombee allow me to get a list of the Most Popular items for either the entire population or a subset of the population?
Yes, we have functionality using the logic parameter for the recommendation endpoints in our API. You can use logic=recombee:popular for this. See more here.
Does boosting work in the opposite direction too if, for example, we wanted to demote recommendations?
If you have some items that you wish to recommend with less probability return e.g. 0.3 for them while you return 1 for the others in the booster.
The minimum is 0 and the maximum is not exactly defined, but the greater number the booster returns, the greater the boost is. You can test which constants work the best according to your preferences.
The minimum is 0 and the maximum is not exactly defined, but the greater number the booster returns, the greater the boost is. You can test which constants work the best according to your preferences.
How many databases can I have?
Unlimited. :) In the Free Plan, the maximum amount of databases you can have is two. If you’d like to have more, we would recommend upgrading your plan.
How can I clear the entire contents of my Recombee database? I was doing some tests and I want to start over.
You can erase all the contents by using the Reset Database option in the settings section of your database in the Admin UI.
Can the solution recommend content based on the user's direct input?
Yes, we can take user’s direct input such as: Preferred categories, Topics of interest, Selected geolocation, Search Query (Semantic/Full-text) into account.
The input can be sent as the user properties (metadata) or with each recommendation request, based on your preference.
The input can be sent as the user properties (metadata) or with each recommendation request, based on your preference.
Can the solution recommend content based on the user's recent views?
Yes, Recombee is a real-time recommender system.
The interaction is processed within a few milliseconds and is taken into consideration on the very next recommendation request.
The interaction is processed within a few milliseconds and is taken into consideration on the very next recommendation request.
Can the solution provide trending content, national and locally?
Yes, as long as we get the geo-information (e.g. as latitude/longitude or a city + state) we are able to provide both national and local trending content.
The geo-information can be provided to Recombee either with each interaction and recommendation request or as a user property, based on your convenience.
The geo-information can be provided to Recombee either with each interaction and recommendation request or as a user property, based on your convenience.
Are recommendations based on the devices the user is coming from?
Yes, we are capable of taking device type into the consideration as slightly different type of content is being consumed across different devices.
Does the engine work in real-time or in batch mode?
A vast majority of the models are fully real-time with incremental training.
Therefore the users can enjoy recommendations that immediately react to their interactions, as well as instant discovery of breaking news.
Models that require batch processing (e.g. neural models that work on top of the article texts and images) are periodically retrained automatically. There is no need to trigger training manually.
Therefore the users can enjoy recommendations that immediately react to their interactions, as well as instant discovery of breaking news.
Models that require batch processing (e.g. neural models that work on top of the article texts and images) are periodically retrained automatically. There is no need to trigger training manually.
Do the clients need to send analytics to the solution?
No, Recombee can compute analytics (such the trending articles) from the received interactions.
How do we maintain the model? Does the model auto-update?
There is no need to manually maintain the models, the models auto-update.
A vast majority of the models are fully real-time with incremental learning.
Models that require batch processing (e.g. some neural models that work on top of the article texts) are periodically retrained automatically.
Recombee thoroughly monitors that the models are up-to-date.
A vast majority of the models are fully real-time with incremental learning.
Models that require batch processing (e.g. some neural models that work on top of the article texts) are periodically retrained automatically.
Recombee thoroughly monitors that the models are up-to-date.
How would the solution handle new content (e.g. breaking news)? Is the content incorporated immediately or on next model update?
The content is incorporated immediately.
Our reinforcement learning models can recognize the breaking news in record time and show them to users at prominent positions within the recommendations.
Our reinforcement learning models can recognize the breaking news in record time and show them to users at prominent positions within the recommendations.
How does your pricing work?
Our standard pricing is purely usage-based. All self-served plans are pay-as-go plans - you never pay more than you use.
The Standard Plan starts at $99/month - you'll get a separate instance on our enterprise infrastructure with high availability guarantees, and API integration support.
The cost of our service is based on four components:
1. number of ingested users’ interactional data
2. number of recommendations requests
3. number of monthly active users
4. number of items in the catalog
When properly integrated, the number of active users is roughly equivalent to the number of users you can find in Google Analytics for a given period of 1 month. For the Recommendation requests, only the number of invoked requests counts, no matter the number of returned items per request.
The Standard Plan starts at $99/month - you'll get a separate instance on our enterprise infrastructure with high availability guarantees, and API integration support.
The cost of our service is based on four components:
1. number of ingested users’ interactional data
2. number of recommendations requests
3. number of monthly active users
4. number of items in the catalog
When properly integrated, the number of active users is roughly equivalent to the number of users you can find in Google Analytics for a given period of 1 month. For the Recommendation requests, only the number of invoked requests counts, no matter the number of returned items per request.
How do you define or constitute an active user?
In Recombee a user is counted as active only if there was at least one recommendation request or interaction (view, purchase, etc.) within the period of one month generated for the user.
What happens after my trial expires? Will I need to pay for anything?
When your trial expires, your account will be automatically downgraded to a Free Plan. You don’t need to do anything. You can always upgrade when needed.
What will I get in a Free Plan?
In a Free Plan, you can have up to 20,000 items in your catalog and your usage should fall under 20,000 active users and 100,000 recommendation requests per month. Please note that the Free Plan option does not contain any support, guarantees, or SLAs.
If you plan to put a Recombee solution into production, we strongly advise you to upgrade to our Standard Plan.
For more info visit this link.
If you plan to put a Recombee solution into production, we strongly advise you to upgrade to our Standard Plan.
For more info visit this link.
I have upgraded my Organization - when will I receive the invoice?
You will receive the invoice one month after the day you upgrade. For e.g. if you upgrade on November 16, you will receive the invoice on December 16th. Recombee charges its clients for the services provided in the previous month/period, thus the invoice is sent after a month’s usage.
I did not log in into my upgraded Organization and use the Recombee services for a full month. Will I still need to pay for the services?
Yes, you will still need to pay for the services, despite not using Recombee’s services for a full month. When a Recombee account is upgraded to a Standard Plan, it is moved to an "upgraded/paid/premium" server which has priority support from our tech team, and guarantees: 99.5% uptime for the recommender, average response time less than 500 milliseconds, and 95% Admin User Interface availability.
Where can I find the necessary documents to start integrating?
You can find the necessary documents here.
What is the easiest way to integrate Recombee?
The simplest method to integrate Recombee involves a two-step process.
- Synchronize your item catalog, learn more here.
- Show the recommendations on your website via the code which is automatically generated by our No-Code widget that is accessible through our Admin UI.
In the case of the Recommend Items To Item scenario, it also automatically sends information about viewing the item (product, article, etc.) by the particular user to Recombee.
How can I invite teammates to my account?
You can invite teammates to your account easily in your Recombee Admin Interface. There is a “Manage Access” button on your “Organization” page. Once you click on it, you can add collaborators and give them specific permissions (what they can or can’t see/do). See more here.
When it comes to sharing our Item catalog with you, is there a preference between using the Recombee API or the Google Merchant feed?
If you already have an existing Google merchant catalog implemented, provided that to Recombee means less work for you. Otherwise, using the API is the preferred way for multiple reasons:
- Full control of the set of attributes and their data types.
- Real-time behavior. While product feed is periodically re-fetched, when using the API, as soon as the data update requests are acknowledged, you can be sure they are used by the models, ReQL, etc.
How can I delete an item from the database?
Please see this link to learn how to delete an item from the database.
However, if the item is just not available anymore, you should rather use a ReQL filter and don’t delete the item entirely, in order to keep the data (mainly interactions) associated with the item for the recommendation models.
However, if the item is just not available anymore, you should rather use a ReQL filter and don’t delete the item entirely, in order to keep the data (mainly interactions) associated with the item for the recommendation models.
Are there limits to the number or size of item properties?
Hundreds of item properties and dozens of kilobytes of texts per item should be fine.The vast majority of our clients fit into these numbers, but we can always do individual adjustments.
How much feature engineering/processing is expected to be done before adding properties to an item?
Providing some structured properties (like categories, tags, etc.) is helpful for both the models and possible ReQL filtering/boosting when requesting recommendations, but plaintext properties are fine. We have NLP models capable of processing raw texts.
I want to deploy Recombee at many websites at once - do you provide an API for automated managing (creating/deleting) of databases?
Yes, we do. Please contact us at support@recombee.com and we will give you all the necessary information.
I want to get recommended items only from a certain category. How do I do this?
You can do it easily using our innovative query language ReQL. Each recommendation request has a parameter filter, where you can specify a condition based on the values of the properties. For example, if you have specified a string item property called category and want to get only books, the filter is ‘category’==”books”. You can also do it in your Recombee Admin Interface.
What is the Data Quality Score on the dashboard?
Data Quality Score is just an estimate based mainly on the fact of whether there are interactions with each of your items.
Recommendations can work flawlessly even if the Data Quality Score is not at 100 %.
Recommendations can work flawlessly even if the Data Quality Score is not at 100 %.
What types of algorithms do you use for computing recommendations?
We use an ensemble of collaborative filtering (matrix factorization, nearest neighbors …) algorithms, which work on the interactions (views, purchases…), and content-based algorithms, which work on the item properties (titles, descriptions…).
The decision about used algorithms is automated - if there is not a lot of interactions (for example in the case of new (cold start) items), content-based algorithms are chosen. In case of a sufficient number of interactions, collaborative filtering algorithms are preferred as they in most cases give the best results. You can learn more here.
The decision about used algorithms is automated - if there is not a lot of interactions (for example in the case of new (cold start) items), content-based algorithms are chosen. In case of a sufficient number of interactions, collaborative filtering algorithms are preferred as they in most cases give the best results. You can learn more here.
How do I evaluate or track the effectiveness of the Recombee recommendation?
In order to get precise success metrics in the Admin UI (how many clicks there were from the recommendations in a particular scenario etc.), it’s good to set optional parameter recommId of the interaction requests whenever the interaction is due to clicking a recommended item. You can learn more here.
What third-party integrations does the solution require?
No third-party integrations are required.
What integration opportunities does the solution provide?
Recombee provides REST API for both receiving data and serving recommendations.
There are SDKs provided for various programming languages for easy integration.
Various feeds can be set up for synchronizing the Items catalog.
We provide an integration with Segment for getting interactions.
Besides the API & SDK approach, a no-code solution is also provided in the form of a No-Code Widget (suitable e.g. for a quick PoC).
There are SDKs provided for various programming languages for easy integration.
Various feeds can be set up for synchronizing the Items catalog.
We provide an integration with Segment for getting interactions.
Besides the API & SDK approach, a no-code solution is also provided in the form of a No-Code Widget (suitable e.g. for a quick PoC).