Breaking the News: The Role of AI in Modern Journalism

Artificial Intelligence (AI) has rapidly transformed the media industry in recent years. From automated news production to trend analysis and personalized content recommendations, AI has brought significant changes to the way media is created, distributed, and consumed.
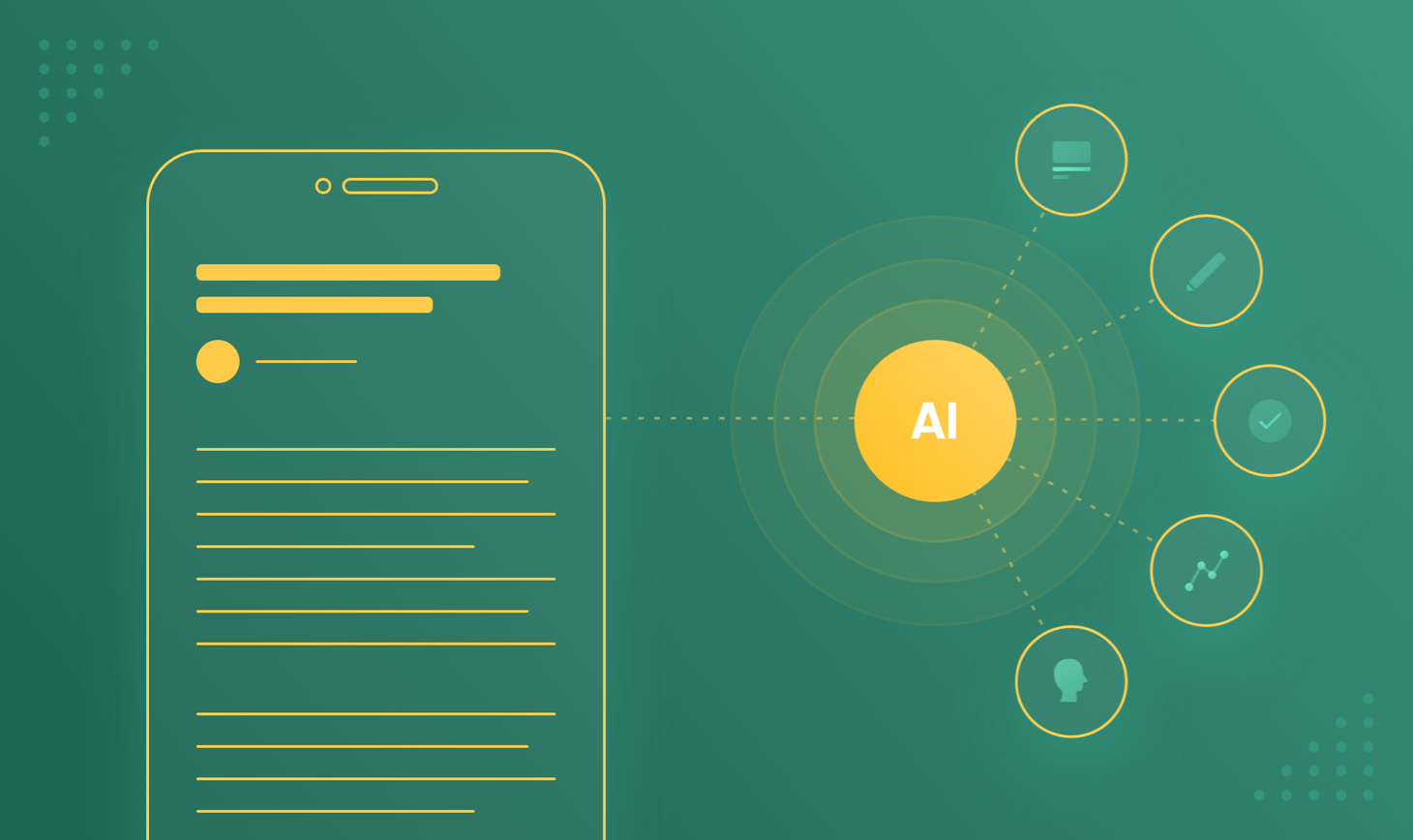
AI algorithms can help news organizations quickly process large amounts of data to identify patterns, analyze and capture trends, verify facts, detect fake news, etc. AI can also generate headlines and illustrations, enrich the article with external sources, convert articles into spoken form and vice versa.
Beyond these assistant tasks, AI can be used to generate content. Simple templated sports or stock market texts, daily summaries of important news in the form of long-form summaries, are generated by modern language models rapidly replacing rule-based AI systems. Moreover, AI can also be used to write texts in any genre on any topic, ready for publication with just a little editorial tweaking. There are several AI writing tools that you can try such as ChatGPT, Smodin Author, Writesonic, Jasper, Article Forge, Ink, AI Writer, Word AI or You.com.
However, AI in media also comes with its own set of challenges and concerns. One major issue is the potential for generative AI to fabricate and amplify misleading and harmful content with the consequence of radicalizing society or manipulating public opinion.
In order to reduce such risks, researchers and engineers have developed tools that can recognize AI generated content which may be inaccurate or even completely fabricated. While some tools are already available such as OpenAI Classifier or Groover from the Allen institute, they are not perfect as the task of identifying AI generated content is much more difficult than, for example, detecting counterfeit items. As generative AI flourishes, detection methods will always be lagging behind and we will continue to rely on experienced fact checkers who are rapidly adopting AI tools.
Another key area where AI is transforming media is content distribution. As the prevalence of online editions of content has risen, people have gained access to a virtually unlimited supply of content. But sorting through all that information can be overwhelming. AI algorithms analyze a person's viewing habits, preferences, and behavior to provide highly personalized content recommendations. This increases user engagement and helps users discover new and relevant content they may have missed otherwise.
In Recombee, we work with a number of public and private media organizations. We provide them with a recommender system as a service which distributes unique and tailored content to their users. We need to be sure that our recommendations are not biased or harmful. In our previous blogpost, we demonstrated how algorithmic biases can be detected and reduced. Another potentially harmful feature of algorithmic recommendation is the emergence of filter bubbles and the negative effect on the audience that might be a bit overstated in mainstream news. Fortunately, our experiments confirm that users prefer not to be locked in the filter bubbles and our algorithms help them to explore. Media companies should be aware of this risk and should avoid using simple collaborative filtering algorithms or content based methods optimized for simple criteria such as the offline recall.
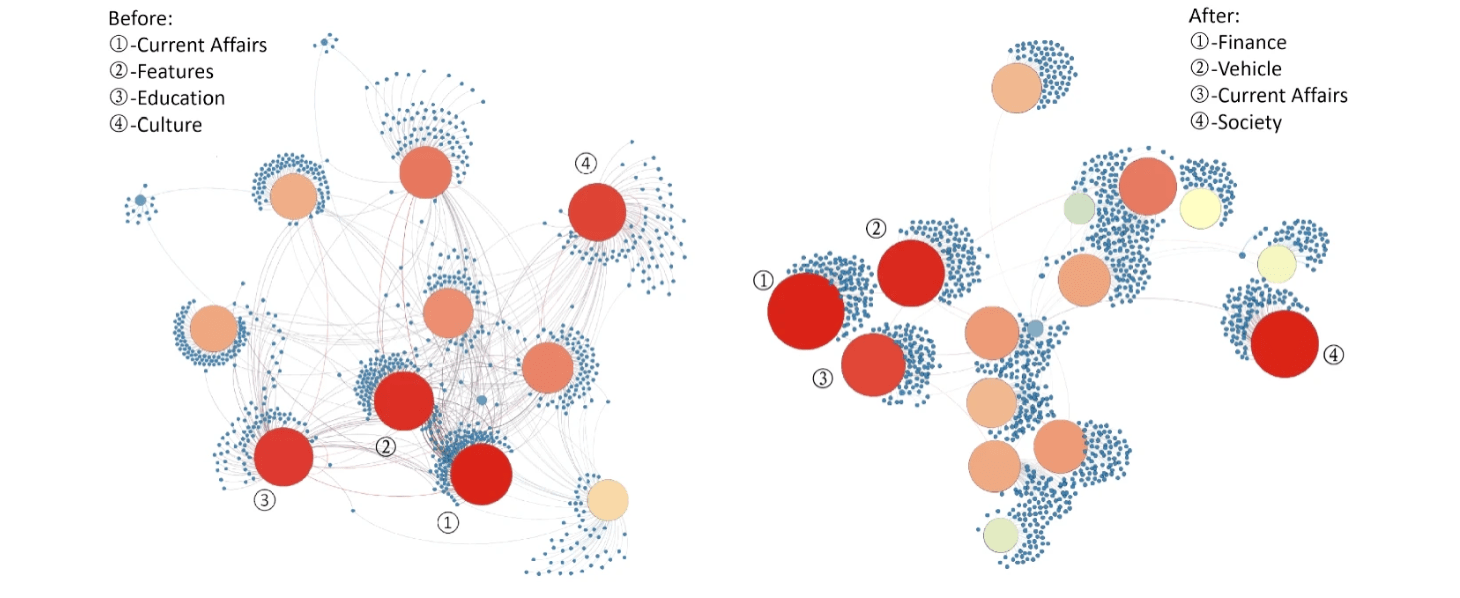
From our perspective, the implementation of AI algorithms in the news industry has had a positive impact. Through the use of personalised recommendation technology, our customers have observed that content producers are able to reach their target audience more efficiently and effectively. This technology allows for niche content, which might not have been prominently featured on the front page, to receive ample attention by connecting with the relevant audience through website recommendations or personalised newsletters.
From Theory to Practice: Insights from Professionals on the Frontlines
We wanted to know how the media organizations across Europe see the role of AI in their environment. That is why we asked media professionals to share their ideas, experiences, and concerns about using AI in the media industry. We are happy to share some interesting thoughts from the very inspiring conversations we had in preparation for the Discussion panel in the AI Mellontology (futurology) Symposium. We paid particular attention to public media, because, by its very nature, it has the most demanding requirements for AI. Since it can still be a sensitive topic, some have asked us to remain anonymous. We respect their wishes and have anonymized their comments using their initials.
As one our correspondent (let's call them J from a big public radio company) points out: We need to clarify and explore “how companies and governments deal with AI and what our ethical guidelines are about it.” K (from a multinational telecommunications company) sums up: “We are not like Netflix.”
AI’s Efficiency
Artificial intelligence is considered a very promising tool, or, more precisely, approach. If we focus only on content, then almost all public media successfully use it for data preparation, automatic content generation, and content distribution. The automatic message location, metadata extraction, and fact-checking save a lot of time. Or it can be used for "automatic song recognition", as mentioned by John Patrick Rott from Hessischer Rundfunk.
“It could help the newsroom get more time and really focus on journalist work. Editors should work on reports where they bring in their view and where we can use their expertise as journalists; since writing normal reports is a little bit of a waste of time” J highlights the benefits of using AI to generate content automatically. On the other hand, this also brings complexities because, as J continues, "We will have to teach people to distinguish between artificially generated text by a synthetic medium and real quality content prepared by a journalist."
Transparency and Responsibility in Recommendation Systems for Public Media
Concerning the distribution of content, public service media, in particular, has very strict requirements for recommendation systems in terms of transparency, credibility, and the possibility of editorial intervention.
Cristina Kadar from Neue Zürcher Zeitung, who joined our panel, impressed us with her presentation. As demonstrated in her presentation slide, the team achieved significantly higher user engagement rates while also prioritizing ethical considerations such as mitigating algorithmic bias and upholding their responsibilities as upholding their high journalistic standards as the Swiss-German newspaper of record.
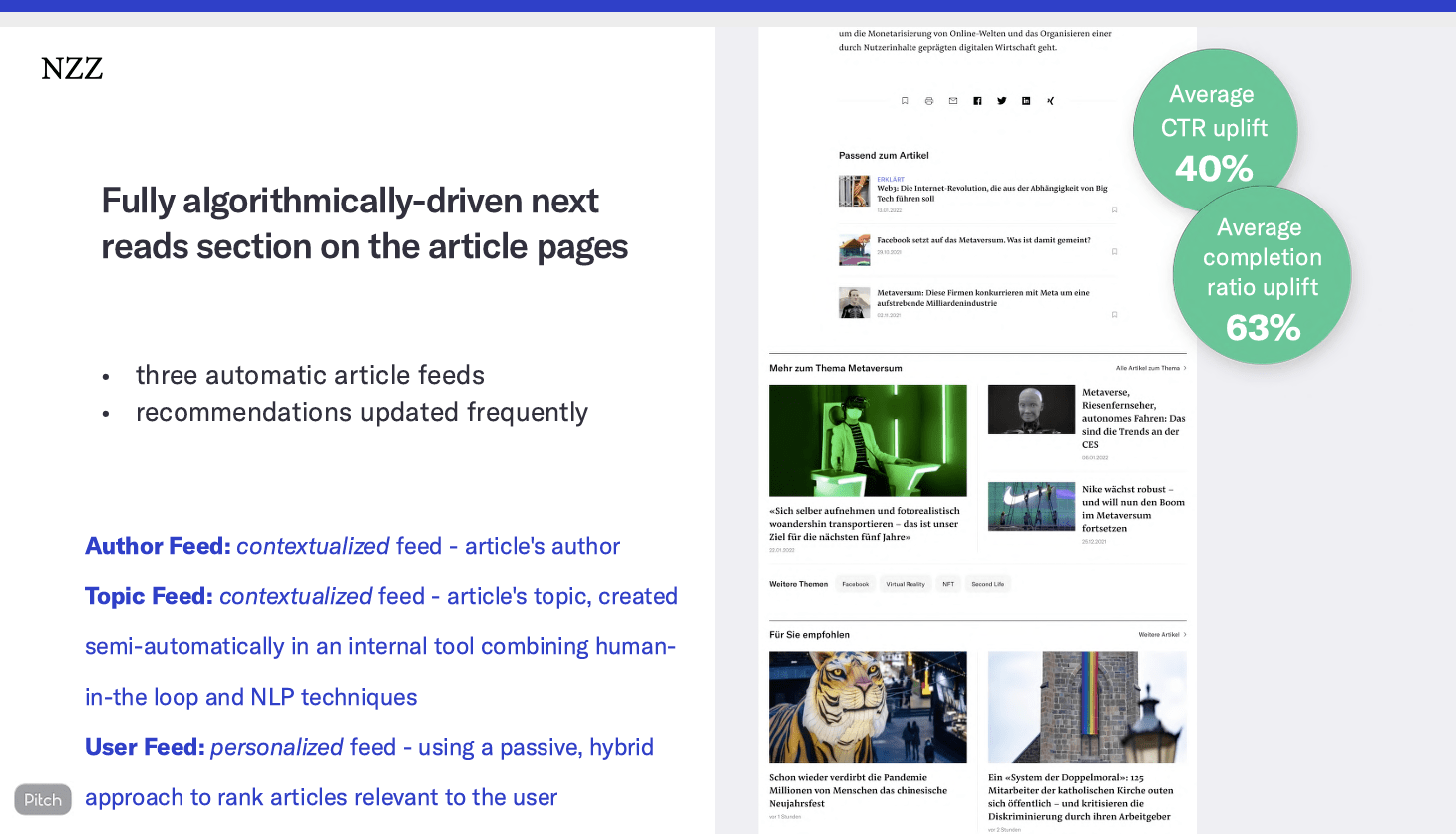
Considerations for User Information and Metrics in Recommender Systems
Very careful consideration must also be given to what user information the Recommender is allowed to use and how to properly evaluate user actions.
“Before implementing a recommender system, it's important to define the actions that will be used to measure success. Will it be based solely on clicks, or should other factors, such as time spent on a page, be taken into consideration?
This can be a challenging task, as it requires careful consideration of what metrics will accurately reflect user engagement and satisfaction with the recommended content”, according to Simone Spaccarotella from BBC.
“The purpose of making data available is not to simply release it without any direction, but rather to use it strategically to motivate people towards a specific goal or direction. It's important to choose Key Performance Indicators (KPIs) that align with the transformation you wish to achieve. By selecting the right KPIs, you can motivate individuals to take actions that will drive the desired transformation and ultimately achieve your goals,” emphasizes F from a big public news organization.
Challenges Beyond AI: Adapting to New Platforms and Changes in Information Consumption
AI is not the only challenge that traditional public service media must face. The development of new platforms and a shift in the way we consume information are also bringing new pressures for change.
“In the past, it was possible to set the agenda and direct users towards content within your ecosystem by simply moving them around. However, with the rise of streaming platforms, the majority of users (approximately 80%) are now making decisions about what to watch before even arriving on the platform. As a result, the role of platforms has shifted from being a leader in content direction to being more of a facilitator in helping users find the content they are already seeking”, concludes J.
When you are not only distributing your content, but also allow third party content to your newsfeed, there are even more challenges involved. Karel Koupil from Seznam pointed out in his talk at AI Mentology Symposium, "After giving third-party content producers access to our homepage, we immediately noticed an influx of click-bait content. In order to maintain the quality of our platform, we had to take swift action to address the issue."
However, eliminating click-bait content completely is also not the best solution. "Many people are primarily seeking entertainment when consuming content, and are often aware that some click-bait content may not offer substantial value. However, the presence of a small portion of such content can actually improve their loyalty and even increase engagement with more serious reporting. This is because a degree of variety can help break up the monotony and provide a more engaging and enjoyable experience for readers. Ultimately, striking the right balance between entertainment and informative content is key to keeping audiences engaged and loyal over the long term." concludes Karel.
These interviews and presentations have been very enlightening for us and we have gained a better understanding of the challenges that not only public relations professionals encounter. Many thanks for that. We do not aim to cover the entire AI ecosystem in media organizations. In our next blog post, we will explain how we can assist editors and media professionals in gaining a better understanding of AI recommendation systems and their readers, enabling them to gain more insight into the produced recommendations. And if you share our interest in the intersection of artificial intelligence and media, we highly recommend delving into this insightful and comprehensive study on the subject.
We are also speaking at the Data Technology Seminar organized by the European Broadcasting Union.
If you are interested in meeting us in person in Geneva please contact us at pavel.kordik@recombee.com or at tana.lancova@recombee.com
Next Articles
Inductive Matrix Completion: How to Improve Recommendations for Cold Start Users and Items by Incorporating Their Attributes
Matrix completion (MC), the problem of recovering the missing entries of a partially observed matrix, has found use in a wide range of domains. Still, its potentially most successful application is as a collaborative filtering technique for recommender systems (RSs)...

Innovative Personalization Features for 2023
The digital world is changing; users' expectations for personalization are increasing, and our Recombee features are continuously improving. One of our focuses is to support our clients in providing the best possible user experiences...

Recombee Item Segmentations
Item Segmentations are Recombee's original and elegant solution to various advanced tasks related to hierarchical and relational data. The feature provides a flexible way to group items (products or pieces of content) into segments...
