AI News and Outlook for 2024

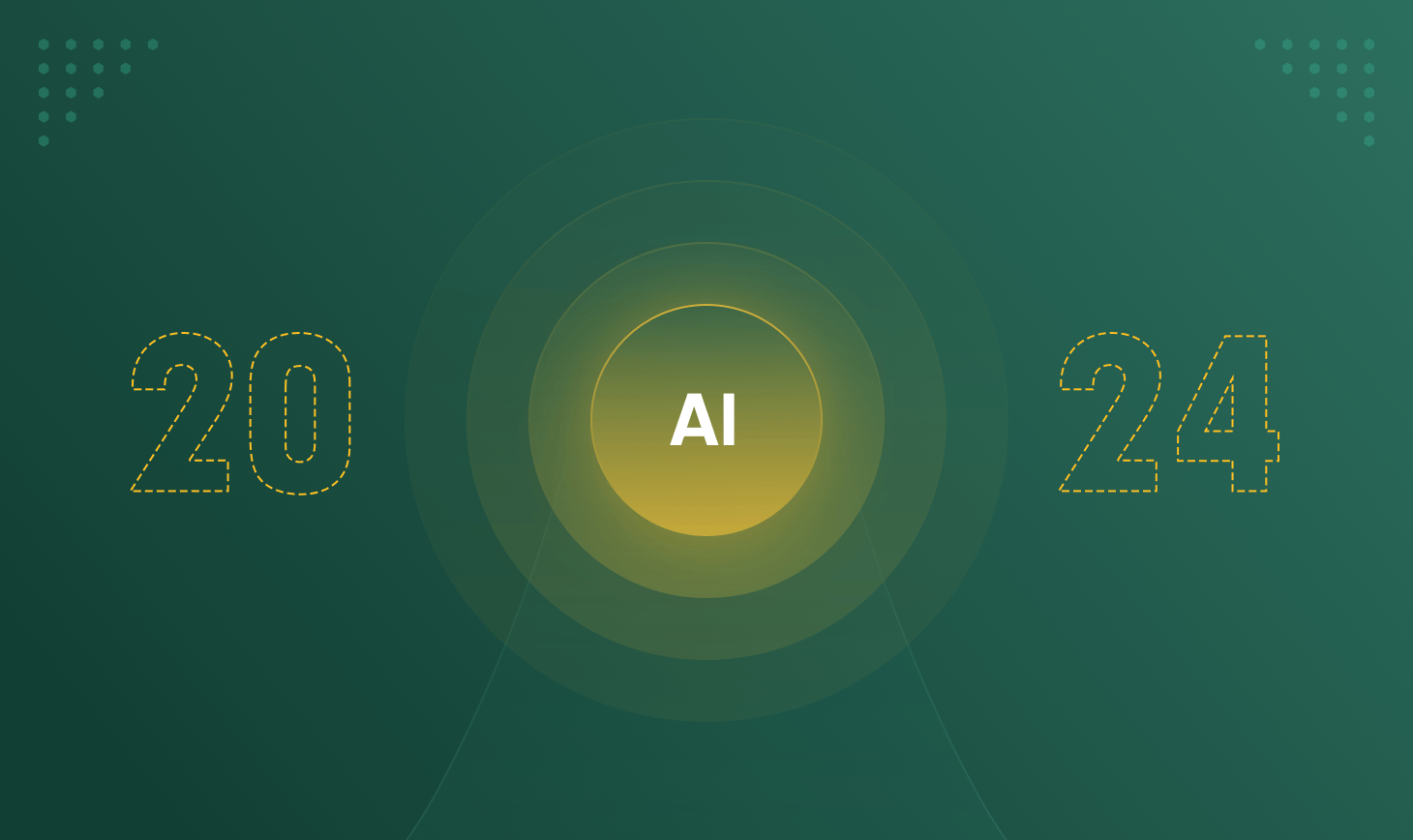
We look at the most interesting research directions and assess the state of knowledge in key areas of AI. We'll also estimate future developments in 2024 so you know what to prepare for.
I have been involved in artificial intelligence research, specifically artificial neural networks, for 25 years. All this time, I have been trying to keep up with the latest trends and approaches, which, especially lately, has become quite challenging given the breakneck pace of development in this field. In this article I try to summarize in an accessible way the most interesting recent discoveries that will have a significant impact on how the world around us will look like. After all, in the field of AI, the time from discovery, to application development, to global adoption is incredibly short. That's why we can expect to see the latest innovations in AI research come to fruition in a matter of months. So if you want to be at least a little bit prepared for what's coming in 2024, read the following.
Transformers in Data Centres
The advent of so-called large language models (LLMs), which we talk to through, for example, ChatGPT or Bing, is due to advances in deep neural network architectures. So-called deep neural autoencoders learn to add words to sentences or reconstruct parts of images on huge datasets. This forces them to create their own internal representation of the world and understand how language, art, music or spoken word works. This is why we may think they are intelligent, even if they are only very good at predicting the following text or generating images from text.
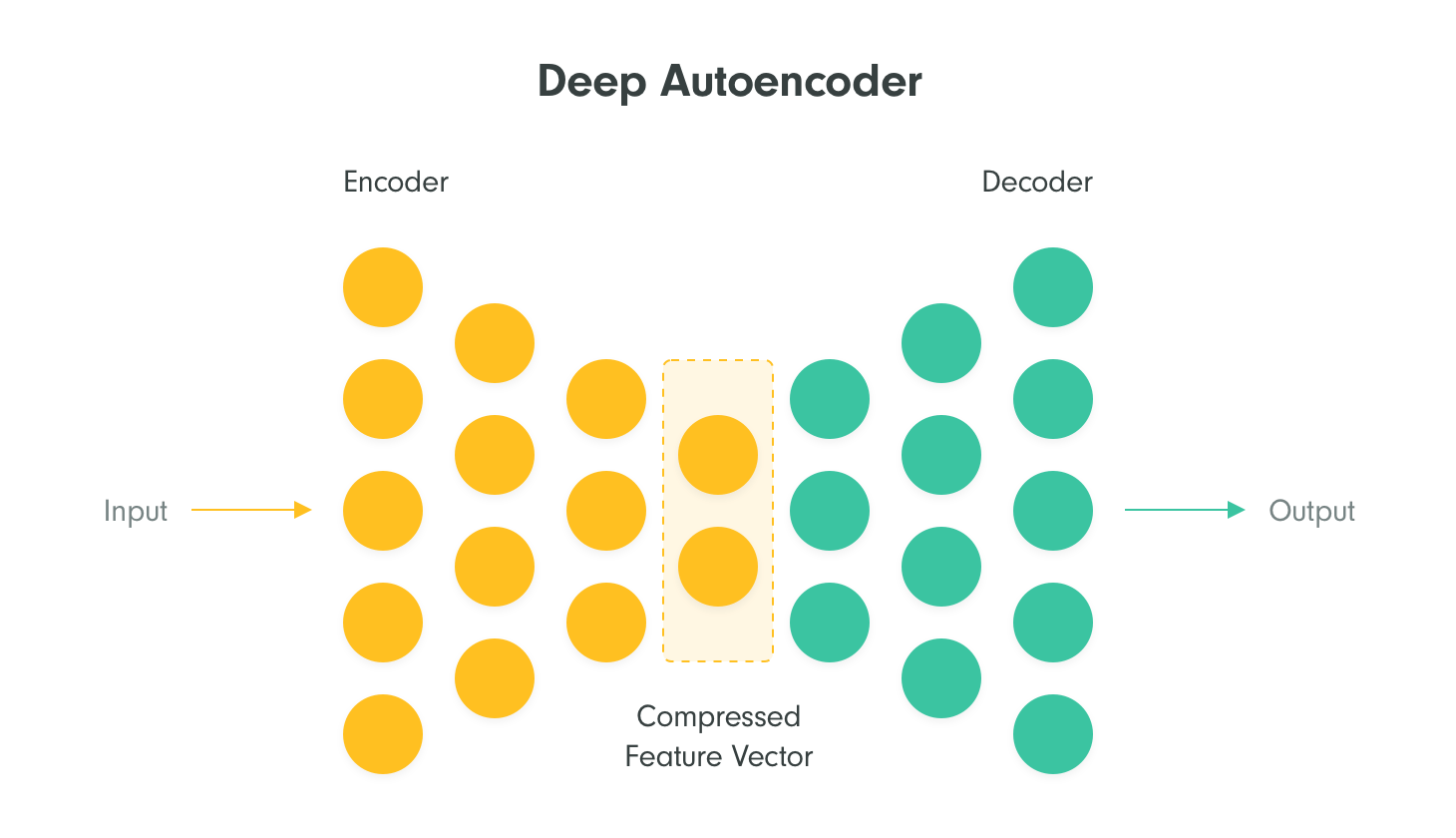
The so-called Transformer is a special neural autoencoder that stores information during learning in so-called attention matrices, which directly express, for example, connections between words. It turns out that when we increase the capacity of the transformer (number and size of maps) the results keep improving. Modern LLMs are therefore trained and run on large computing infrastructures.
Economics of AI Products
There is a cost to training and running large models. Despite all the optimizations, running good LLM models is still quite costly. That's why only large corporations like Microsoft, Google or Amazon offer them for free and make a lot of money. As with other AI products, they are looking for a way to make money from running them. Interestingly, there aren't many production AI systems yet, other than recommendation and search algorithms, that pay real money to run. But it seems that users are willing to pay for access to good language models and services built on top of them, because it will pay them back. I think that this year will see the emergence of a lot of companies offering customers subscription-based time-saving services built on top of LLM. Both cheap ones for the mass market (ala ChatGPT Plus or Gitlab Copilot) and more expensive ones that add a lot of value for a small group of users. And this is where there is huge potential, while at the same time not being in such danger from global players.
It's easy to build a service on top of ready-made models, you just need to think about what your competitive advantage will be. Or you can run your smaller language model locally, even on mobile phones, so applications can work even without internet access. Of course, the capabilities of these models, especially the smaller ones, are quite limited. Let's now take a look at how they could be improved this year.
What Is in Store for Us This Year?
Of course, it is difficult to estimate given the pace of development, but I will at least try to make a rough prediction.
Although LLMs are starting to be used as reasoning engines, it is their limited ability to reason analytically that is their biggest weakness. Here we can expect LLMs to be enriched with technologies we know from decades of reinforcement learning research, and AI assistants to maintain their model of the world. They can predict future events, use advanced attentional mechanisms to track important changes and perform actions based on them. This will bring them closer to how humans operate. Here are some interesting directions (1, 2, 3, 4).
While LLMs can store a lot of information in their weight matrices, because they function similarly to biological neural networks, they are often inaccurate in their equipment. However, many tasks require accurate memorization and fitting of information, and therefore, e.g., LLMs with additional memory are being investigated, which can then function similarly to a Turing machine and thus can solve more complex tasks in the future.
In addition to transformers, promising new neural autoencoder architectures such as SSMs or diffusion models are also emerging.
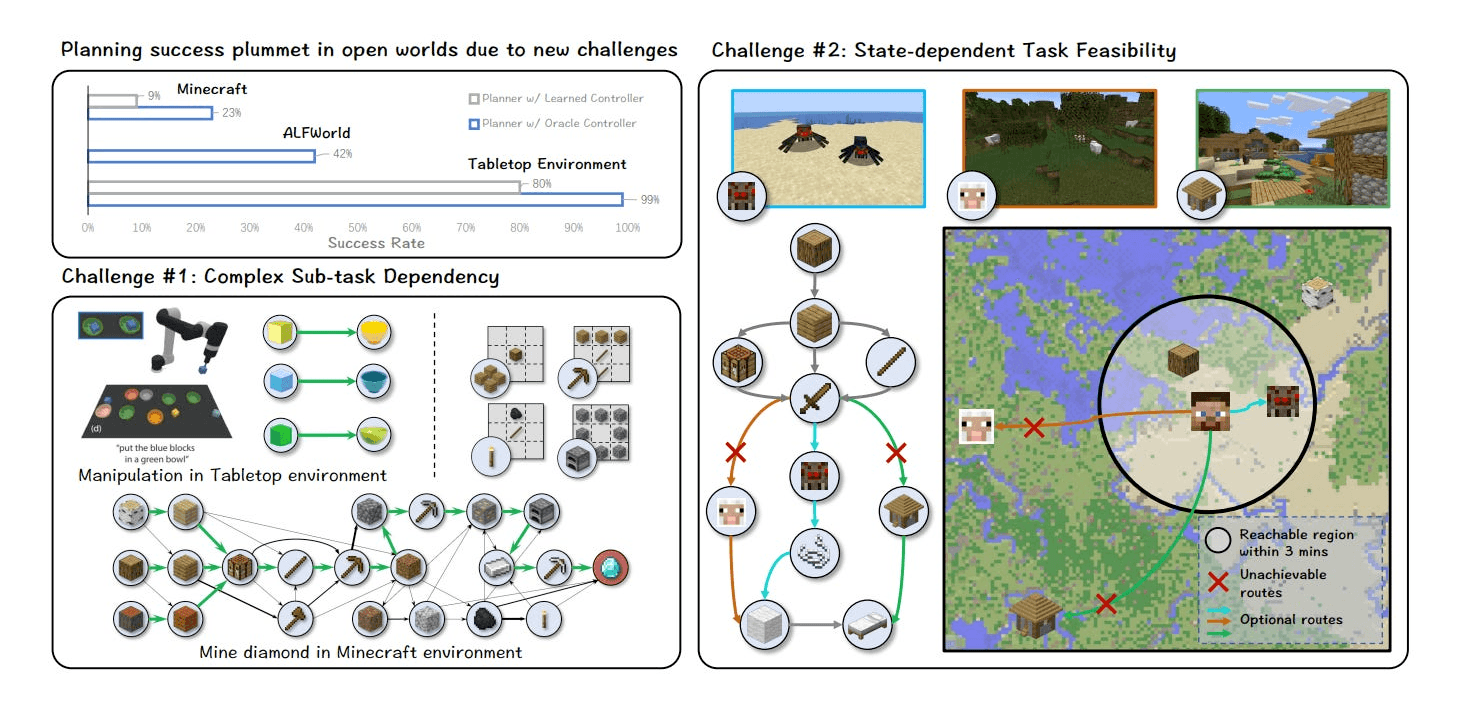
The ability to plan and generalise the acquired knowledge to solve new unfamiliar tasks and the ability to solve multiple tasks in different domains also becomes a very important aspect.
The way in which people can convey their preferences to the models is also improving, thus improving the generated texts and images. Or perhaps to perform more complex robotic tasks.
Being able to measure how well models perform is crucial for AI model research and development. This is very difficult in the case of general AI, so the development of so-called benchmarks will be very helpful. These are of course also essential for the development of self-driving cars, for example. Sometimes it is even useful for the AI to generate the tasks on which it improves itself.
Well, and since all this requires a lot of computing resources, there will be a lot of work on how to make both hardware and especially software faster and cheaper (see LoRa, QLoRa, Flash Attention).
Thanks to all these advances, we will soon have an army of different digital personas and assistants at our disposal. They will no longer be passive, but proactive. I reckon that those of us who pay for more and better assistants in the future will be more efficient, they will make more money because their assistants will be advocating for their interests in the digital world.
Of course, the assistants will be connected to the Internet and will improve themselves by getting real-time information.
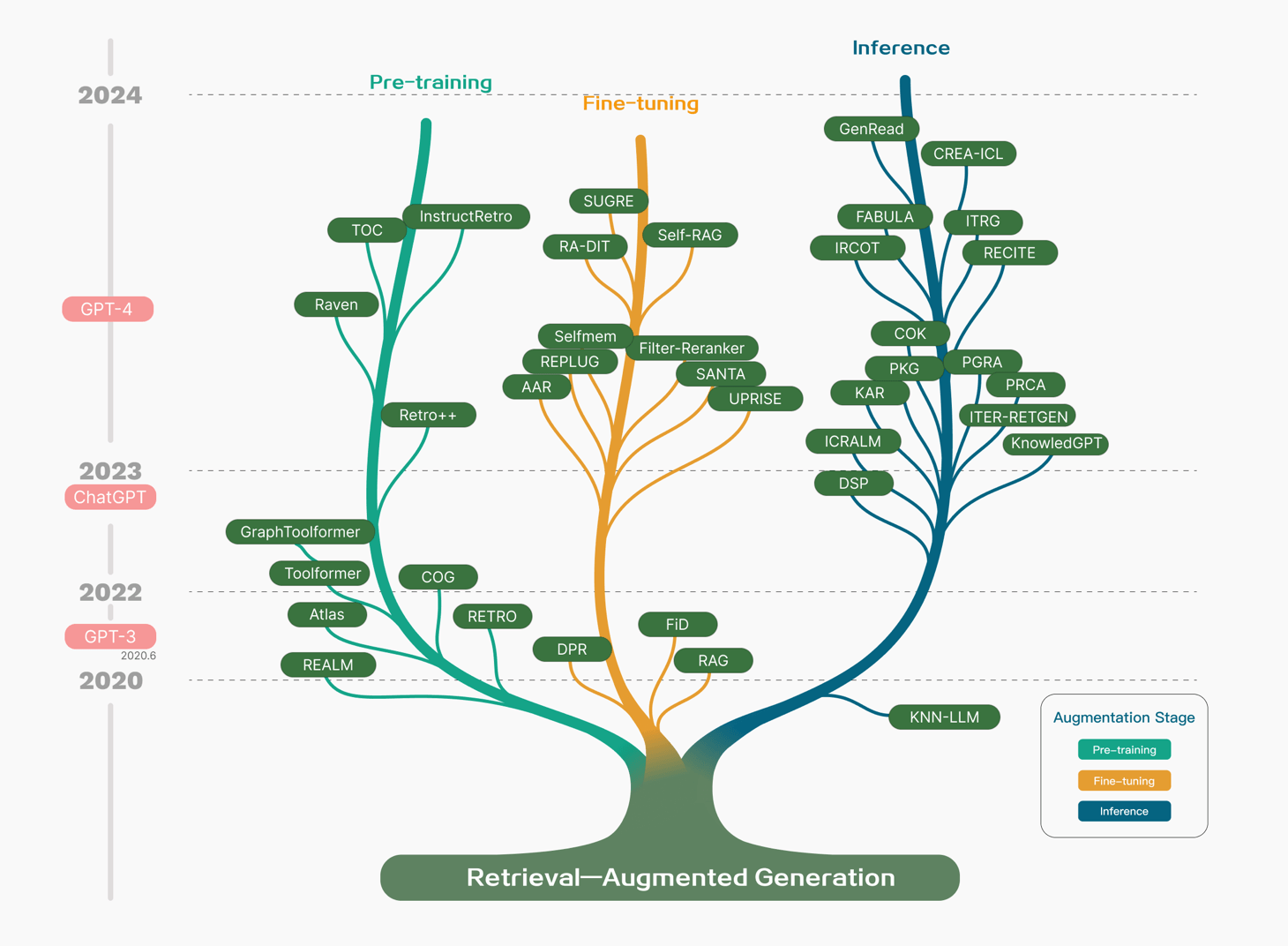
They will also iteratively improve their outputs and actions, similar to the inputs and training datasets. Those who have quick access to new validated information and quality data repositories will be ahead of the game.
Of course, it will also be about finding the most effective interface between humans and AI. Not everyone will be willing to have Elon make a neural implant. And humans are not yet very willing to communicate with machines even in natural language. Maybe it's because the machines haven't understood us for so long. Either way, our recent experience suggests it's probably gonna take some time. At Recombee we have trained a simple LLM model for a US client for a service where you get a recommendation just by asking in natural language. Although the service has found loyal users, the mainstream probably won't end up being impressed in this form. And so we need to experiment with other ways in which new technologies can make our lives more efficient, and hopefully also better.
I wish everyone well for the year of the "full-blown technological AI revolution". I believe it will bring more good than bad to humanity, and that we all can contribute to it too.
Next Articles
Recombeelab's 2023 Research Publications
Recombeelab, a joint research laboratory of Recombee and the Faculty of Information Technology at the Czech Technical University in Prague, experienced a highly productive year in 2023, publishing a series of insightful and impactful papers in the field of recommendation systems.

AI Assistants Know Your Preferences, Even Better Than You Do
Recommender systems and ethical controversies

The AI (R)Evolution in the Media Industry
In today's digital age, personalization has become the cornerstone of the media industry. Whether it's tailoring content recommendations, refining marketing strategies, or enhancing user experiences...
